10. Dimensionality Reduction with Principal Component Analysis
many dimensions are redundant and can be explained by a combination of other dimensions.
Principal component analysis (PCA), an algorithm for linear dimensionality reduction.
PCA - basis / basis change // projection // eigen-values // Gaussian distribution // constrained optimization.
10.1 problem setting.
we are interested in finding projections x ̃ of data points x that are as similar to the original data points as possible and lower intrinsic dimensionality. squared reconstruction error ∥x − x ̃ ∥2 between the original data x and its projection x ̃ . nn
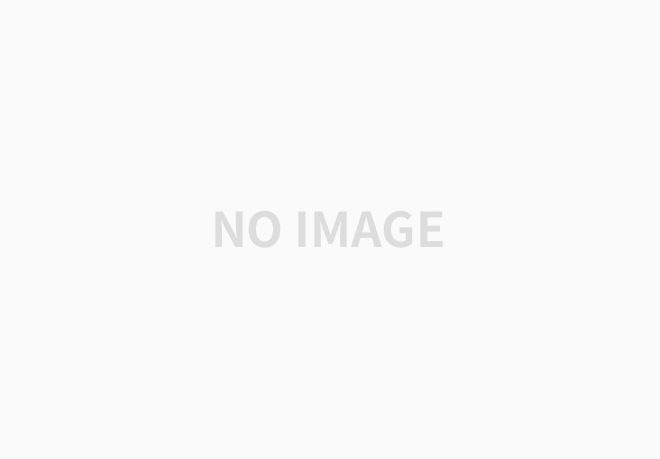
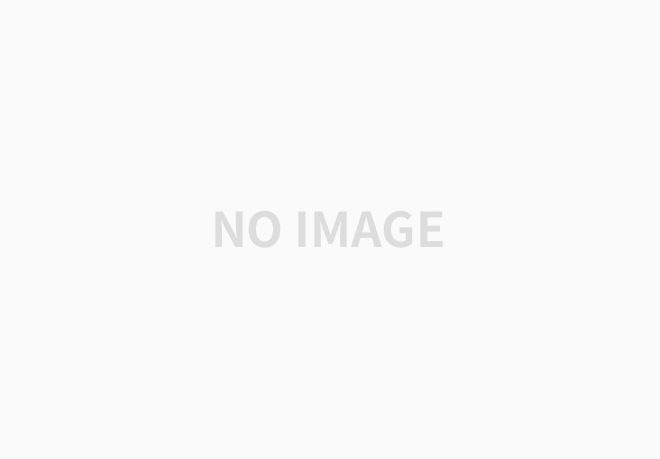
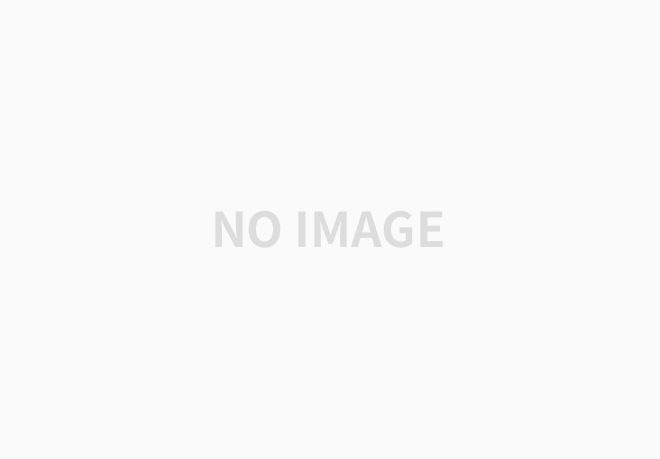
10.2 Maximum variance Perspective
In Section 10.2, we will find low-dimensional representations that retain as much information as possible and minimize the compression loss. ( then we can maintain our information - Key : information )
PCA - Dimensionality reduction algorithm ( maximaizes the variance - for retaining as much information as possible )
10.2.1 Direction with Maximal Variance
maximize the variance of the first coordinate
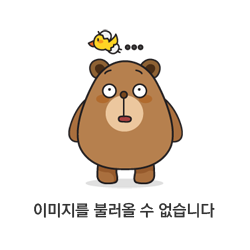
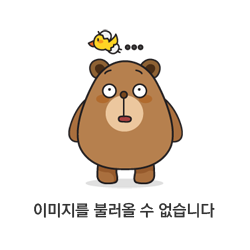
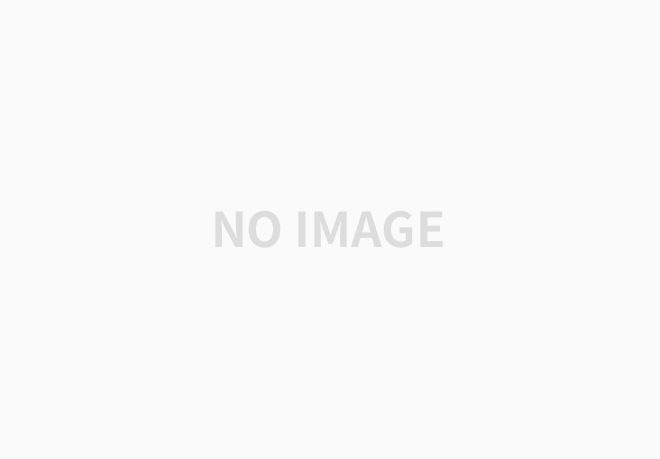
Therefore, we restrict all solutions to ∥b1∥^ 2 = 1, which results in a constrained optimization problem in which we seek the direction along which the data varies most.
b1, b2 ... all vectors are unit vector with restriction to the solution space.
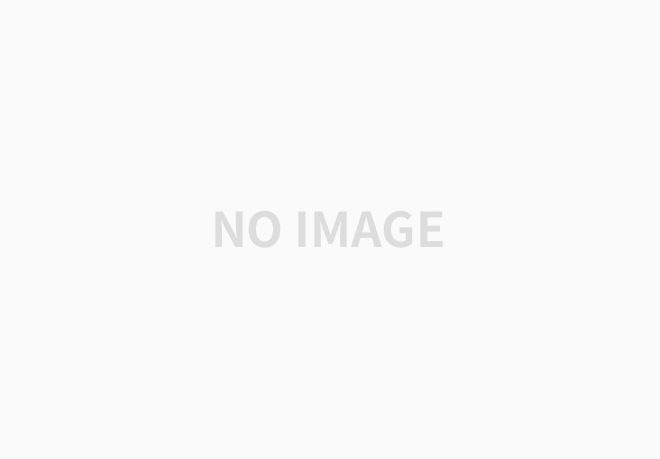
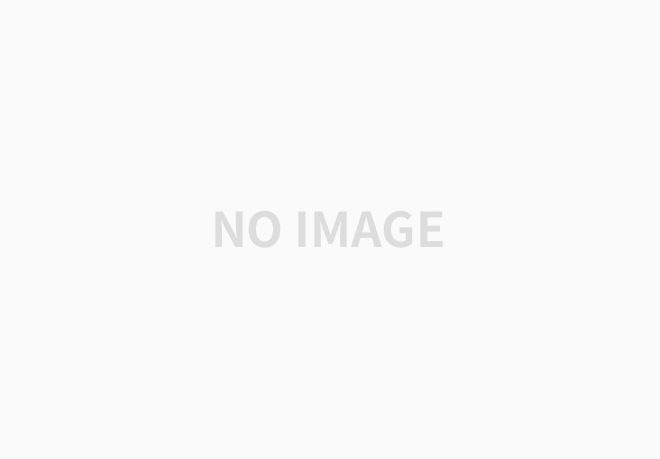
the basis vector associated with the largest eigenvalue of the data covariance matrix.
it is called "first principal component."
b1 - eigenvector of the data covariance matrix S,
Lagrange multiplier - corresponding eigenvalue.
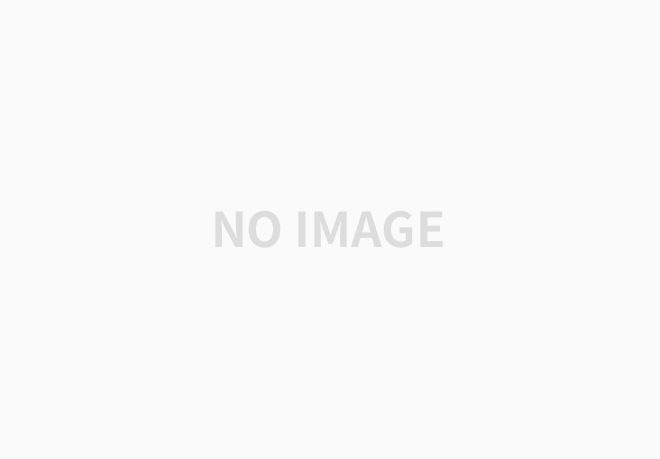
10.2.2 M-dimensional Subspace with Maximal Variance
M th principal component can be found by subtracting the effect of the first m − 1 principal components b1,...,bm−1 from the data.
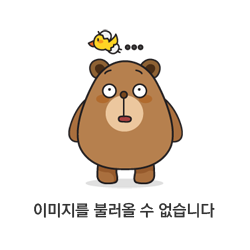
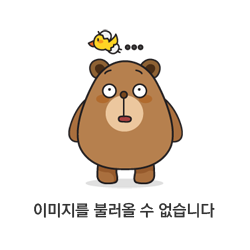
bm is the eigenvector of S^ that is associated with the largest eigenvalue of S^.
bm is also an eigenvector of S.
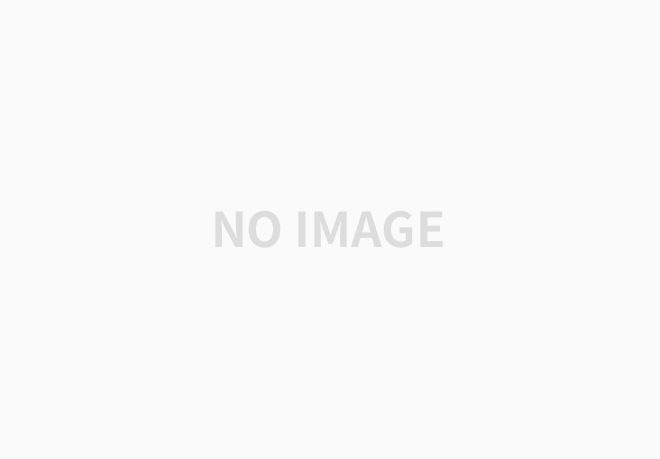
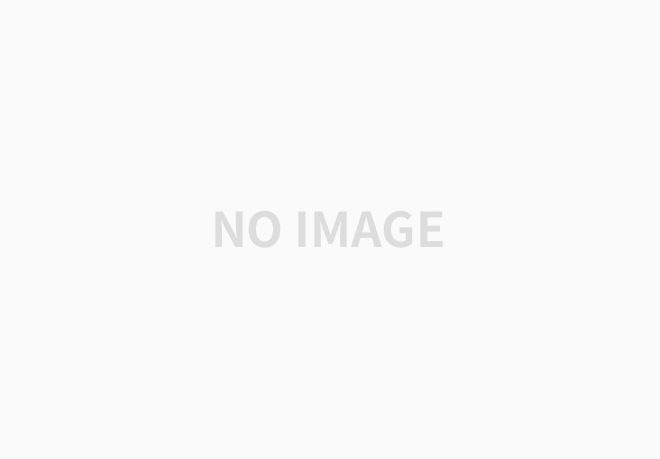
'AI > 북 리뷰 - Mathematics for Machine Learning' 카테고리의 다른 글
11. Density Estimation (0) | 2020.01.27 |
---|
댓글